
The economy may be in recession, but the business intelligence (BI) platform market is still on pace for growth over the next three years

With shrinking IT budget and projection for rest of 2009 looking bleak, organizations need to look into dysfunctional processes that have torpedoed many Business Intelligence (BI) efforts in the past. As BI is driven by data, BI based business decisions should consume data of high integrity. Bottom line - BI (Business Intelligence) for tomorrow need to be moving away from disparate tools and island of data, into an "information-centric" enterprise.
Vendor packages - Oracle Corp, Microsoft, IBM, SAP,.. come with a big promise BI solution. But this often results in ending up with multiple BI solutions in organization's infrastructure that don't work well together, and the quest for "The BI solution" that gives you a consolidated view of all your processes and transactions remains a mirage.
Remember, there may not be perfect data management to start on BI project. I have experienced in the past, Data management is complicated because data in most large enterprises are not organized appropriately or atomic enough to generate appropriate reports consistently. But data without effective data management is rarely information in the larger sense. Poor data quality leads to poor decision
1. Quality of Data: Organizations must establish processes or set of automated controls to identify data quality issues in incoming data and block low-quality data from entering the data warehouse. Implement the process with automated audit trails and alerting using tools, custom code, data quality technology, data integration tools or a combination of these to achieve optimal ETL (Extraction Transformation and Loading) function.
2. Semantics of Data
Semantics data layer should contain the appropriate granularity and derived data based on the usage needs. However, ensure the data in data warehouse is at the most atomic / granular as possible for derivation, but all of this data should not be then made available in semantic layer for BI tools to function. At the same time maintain data definition and derived data consistency. Derived data should have a definition and owner assigned. Ensure to review the data ETL with wrt Business Process with the users, so that they are participants in the journey and not just IT driven activity. Another important participant in the semantics layer is master data. This non transactional data refered as Master data e.g. Customers, Products, Sales Region, Sales Channels, Suppliers, Manufacturers, e.t.c. should be defined with appropriate attributes and consistency for the best value for BI deployment.
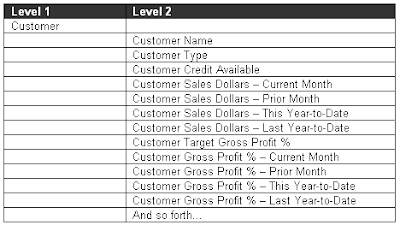
3. Usage Driven Data Model: The goal is to resolve all the semantic differences before designing the data structures for the data warehouse. Whipping data into shape means having expert data modelers spend most of their time working with the business users who understand how the data is intended to be used. Data Models in Semantic layer should be optimized for usage, but remember one shoe can not fit all. If required effort should be made to provide subject area based or user community based semantic models to ensure consistent consumption of data for intended Audience to reach the desired information. Also note, data modeling with home work done on usage pattern using Technology & methodologies plays an important role in deriving how to segment the data for different type of users like Executives, Management, operational team and analysts for their optimal use.
4. Traceability to lowest level
Remember, data quality cannot be solved by the IT department with a tool. Business users must be engaged to determine what is "good enough" in terms of data quality. And don't mistake data accuracy for relevance. That leads to the next big surprises. For all listed data elements, trace them back to their source system(s) and document what transformations they went through before they were printed on the report, displayed on the screen, or written to the extract file. This is where the hidden semantic differences are revealed. Two reports, maybe for two different departments displaying Net Sales, are found to use slightly different calculations-and of course the numbers don't match-how could they? It's found that data on two different reports, while generally identical, is sometimes different. The tracing exercise may reveal that one report is sourced from an unadjusted file and the other report is sourced from the same file, but only after twice-yearly adjustments are made.
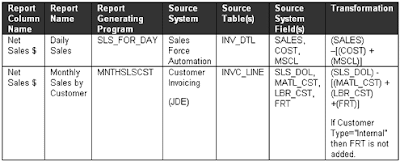
Review the completed element list with definitions and traced mappings with the business users and validate that the document is a complete and correct semantic representation. Facilitate joint sessions with the business users and IT to develop and come to consensus on new terms as needed-e.g. "commission" sales. On the other hand, it may be more appropriate to redefine one or more transformation formulas to conform dissimilar elements. Lastly, base hierarchies does not often resolve the data navigation issues, hence derived dimension should be a key consideration.
5. Strong drivers: BI deployment without strong driver by leadership team will not achieve acceptable results. The biggest problem with BI has always been getting the business teams to agree on what can be made into common definition. From an infrastructure perspective, it is important to try to normalize that relationship between IT and Business, but it's also important to have capable leaders are participating to guide the the IT team vs leaders driving what is right for the management. Similarly, Business manager driven by numbers, but keeping them close to the heart also causes challenges to reconcile the definitions and management. They don't want to have transparency by a dashboard or scorecard or report. Transparency correlates with better business performance. Strong business sponsors who believe in a fact-based approach to management and IT people who can map agreed-upon metrics to the data.
6. Vendor Selection: There is plenty of pressure to go with the standard vendor. The CFO who authorized millions of dollars for ERP may balk at spending more for BI and corporate performance management tools. But "one-stop shopping" doesn't necessarily lower the total cost of ownership or deliver the best fit for your enterprise's needs. Determination of which BI functions are delivered by your enterprise application vendor and compare these functions with those offered by other BI system vendors. Perform evaluation to arrive at the results, often phase zero is better suited before large expense on BI deployment. What ever tool is selected for deployment, do not develop custom functions to the extent that they become high cost of ownership.
In order to be effective with all the mentioned points, you again have to have data management to go along with it and maintain transparency, and BI is a good way to inform that effect, to tell you where redundancies are and study the state of your data.
No comments:
Post a Comment